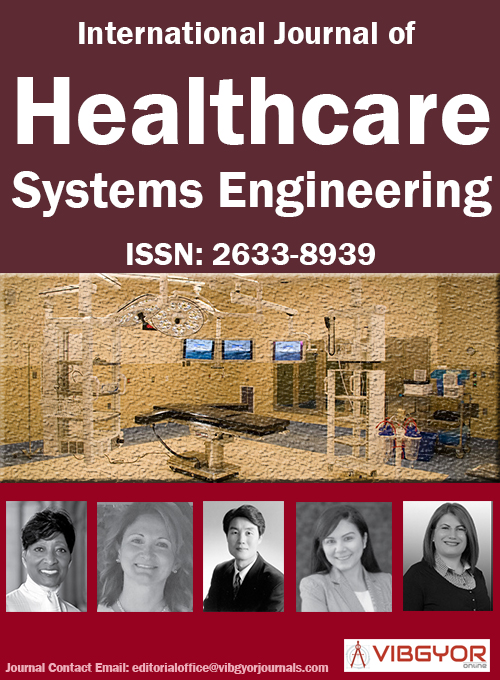
International Journal of Healthcare Systems Engineering
(ISSN: 2633-8939)
Volume 1, Issue 1
Research Article
DOI: 10.35840/2633-8939/7201
Designing Dementia Caregiver Empowerment Interventions through a Simulation: A Conceptualization Study
Rohith Nama1*, Tonya Smith-Jackson1, Younho Seong1, Matthew McCullough1, John Lach2, Azziza Bankole3, Martha Anderson4 and Temple Newbold3
Table of Content
Figures
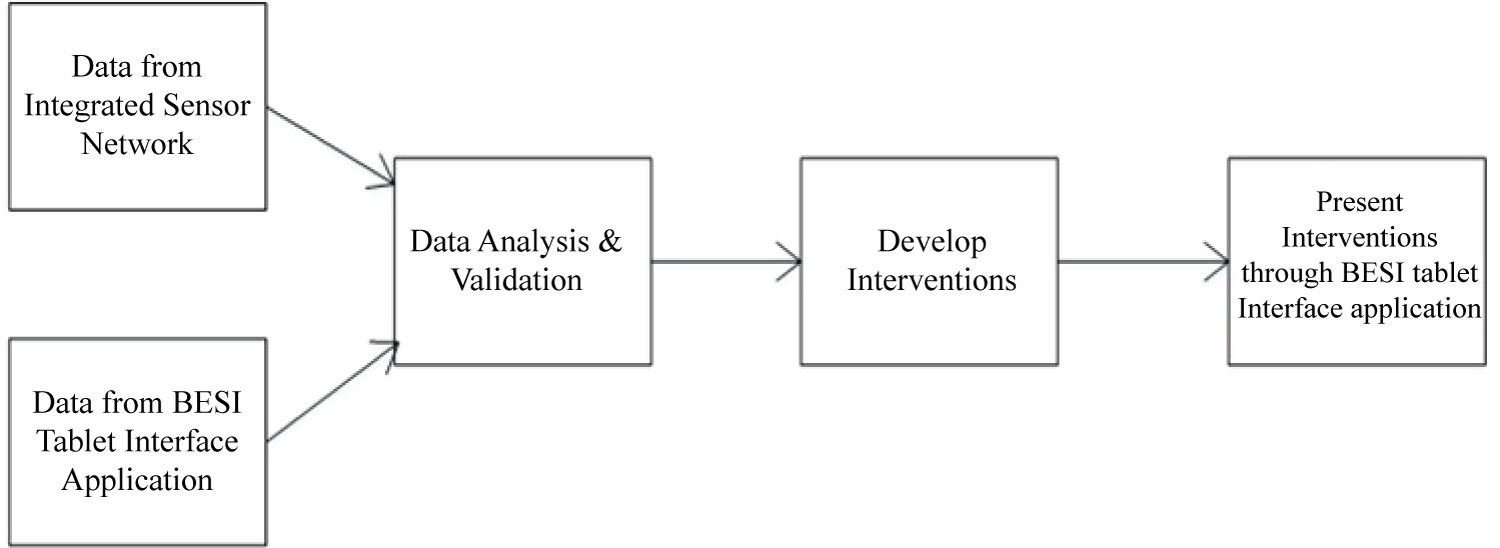
Figure 1: Process of developing and presenting...
Process of developing and presenting interventions to caregivers in the simulated environment.
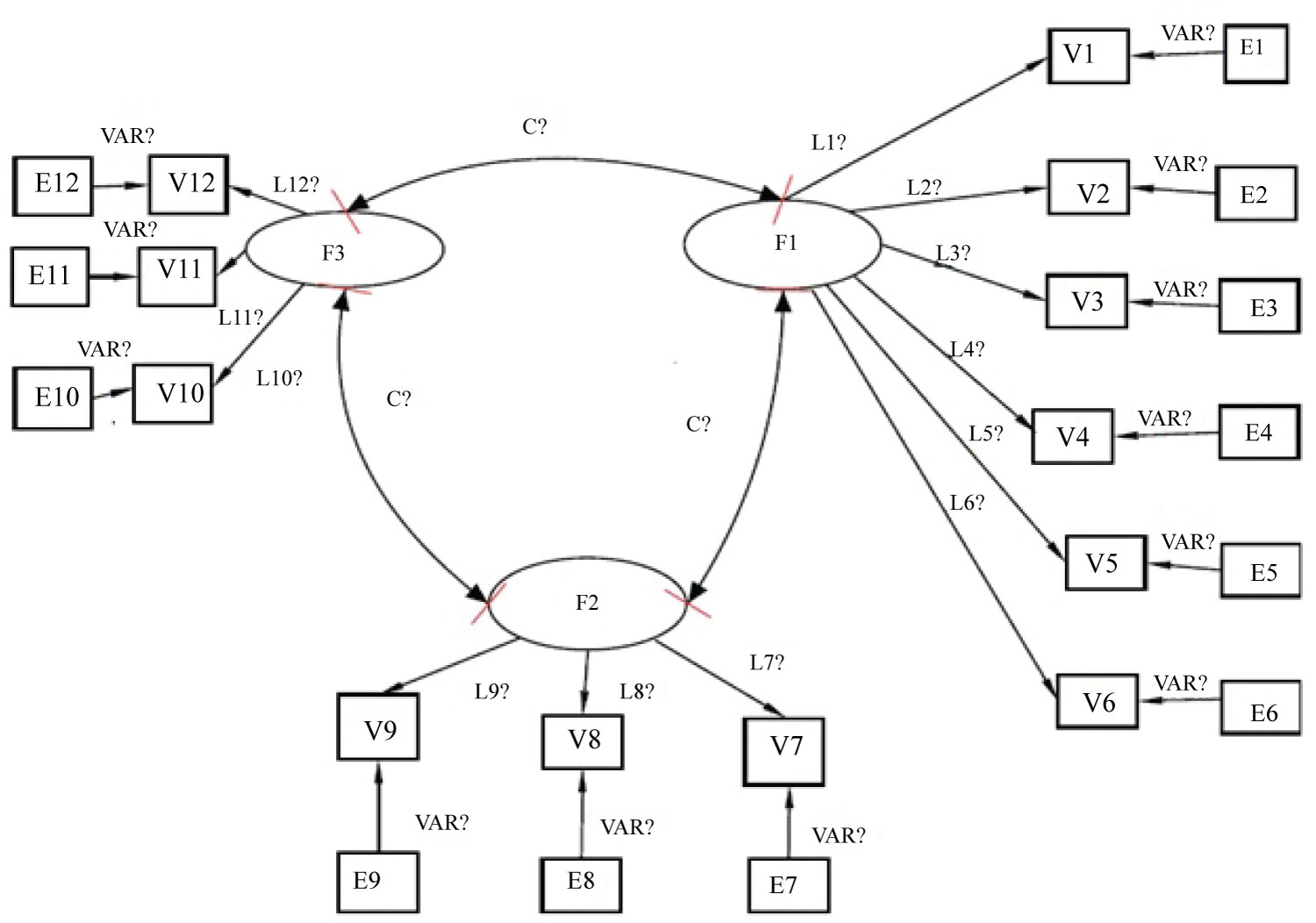
Figure 2: A structural equation model...
A structural equation model to develop inclusive interventions.
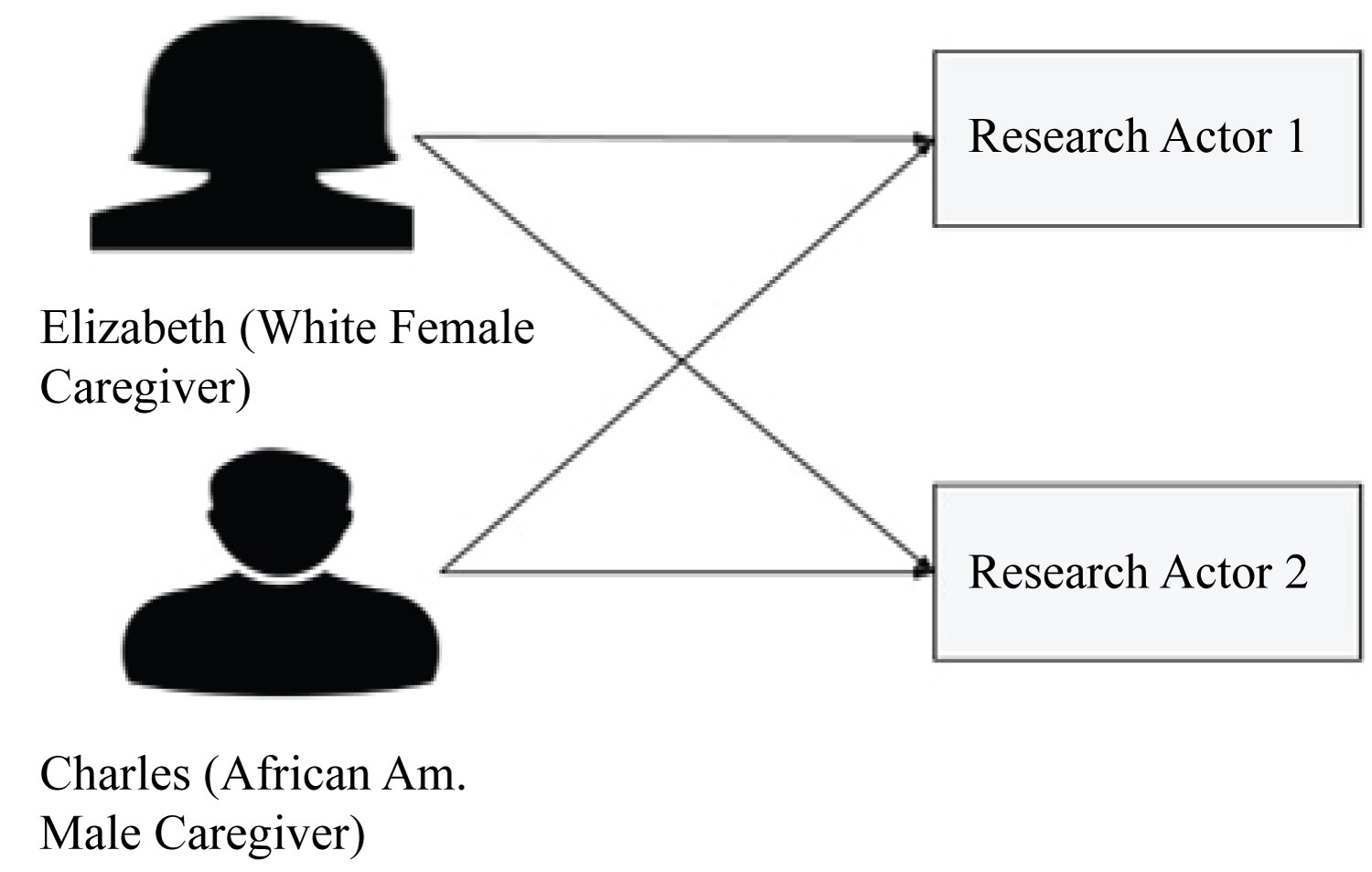
Figure 5: Conceptual data modeling plans...
Conceptual data modeling plans to observe cross-cultural pattern.
Tables
Table 1: List of hypotheses tested using the structural equation model.
Table 2: Manifest and Latent variables used to Develop Interventions.
Table 3: Sensor types used in the BESI research by the collaborative team at UVA.
Table 4: Attributes of a White female caregiver persona.
Table 5: Attributes of an African American male caregiver persona.
Table 6: Confirmatory factor analysis results indicating factor loading for data simulation method 1.
Table 7: Confirmatory factor analysis results indicating the correlation among latent variables for data simulation method 2.
Table 8: Fit indices obtained for confirmatory factor analysis for actor 1.
Table 9: Confirmatory factor analysis results indicating correlation among latent constructs for actor 1.
Table 10: Confirmatory factor analysis results indicating correlation among latent constructs for actor 1.
Table 11: Fit indices obtained for path analysis for actor 1.
Table 12: Path analysis results for actor 1.
Table 13: Fit indices obtained for confirmatory factor analysis for actor 2.
Table 14: Confirmatory factor analysis results indicating factor loadings for actor 2.
Table 15: Confirmatory factor analysis results indicating correlation among latent constructs for actor 2.
Table 16: Fit indices obtained for confirmatory factor analysis for actor 2.
Table 17: Path analysis results for actor 2.
References
- American Psychiatric Association (2013) Diagnostic and statistical manual of mental disorders. Arlington: American Psychiatric Publishing.
- Wilson RS, Segawa E, Boyle PA, Anagnos SE, Hizel LP, et al. (2012) The natural history of cognitive decline in Alzheimer's disease. Psychology and aging 27: 1008.
- Barker WW, Luis CA, Kashuba A, Luis M, Harwood DG, et al. (2002) Relative frequencies of Alzheimer disease, Lewy body, vascular and frontotemporal dementia, and hippocampal sclerosis in the State of Florida Brain Bank. Alzheimer Dis Assoc Disord 16: 203-212.
- Prince M, Wimo A, Guerchet M, Ali G, Wu Y, et al. (2015) World Alzheimer Report 2015. The global impact of dementia. An analysis of prevalence, incidence, cost and trends. Alzheimer's Disease International, London.
- Alzheimer's Association (2015) Alzheimer's disease facts and figures. Alzheimers Dement 11: 332-384.
- WHO (2013) Dementia fact sheet N. 362 2012.
- Allegri RF, Sarasola D, Serrano CM, Taragano FE, Arizaga RL, et al. (2006) Neuropsychiatric symptoms as a predictor of caregiver burden in Alzheimer's disease. Neuropsychiatr Dis Treat 2: 105.
- Jablonski R, Gilmore-Bykovskyi A, Baker N, Bossen A, Molkina D, et al. (2013) Non-pharmacological interventions to reduce agitation in persons with dementia: Considerations for feasibility and future research. Gerontologist.
- Dunkin JJ, Anderson-Hanley C (1998) Dementia caregiver burden: A review of the literature and guidelines for assessment and intervention. Neurology 51: S53-S60.
- Shin IS, Carter M, Masterman D, Fairbanks L, Cummings JL (2005) Neuropsychiatric symptoms and quality of life in Alzheimer disease. Am J Geriatr Psychiatry 13: 469-474.
- Haley WE, Roth DL, Coleton MI, Ford GR, West CA, et al. (1996) Appraisal, coping, and social support as mediators of well-being in black and white family caregivers of patients with Alzheimer's disease. J Consult Clin Psychol 64: 121.
- Connell CM, Gibson GD (1997) Racial, ethnic, and cultural differences in dementia caregiving: Review and analysis. The Gerontologist 37: 355-364.
- Calasanti T, King N (2007) Taking 'women's work' 'like a man': Husbands' experiences of care work. The Gerontologist 47: 516-527.
- Bankole A, Anderson M, Knight A, Oh K, Smith-Jackson T, et al. (2011) Continuous, non-invasive assessment of agitation in dementia using inertial body sensors. Proceedings of the 2nd Conference on Wireless Health.
- Chen S, Gong J, Lach J, Goldman MD (2013) Enhanced multiple sclerosis gait assessment using inertial sensors. Wireless Health.
- Cohen-Mansfield J, Marx MS, Rosenthal AS (1989) A description of agitation in a nursing home. J Gerontol 44: 77-84.
- Alexopoulos GS, Abrams RC, Young RC, Shamoian CA (1988) Cornell scale for depression in dementia. Biological Psychiatry 23: 271-284.
- Buysse DJ, Reynolds CF, Monk TH, Berman SR, Kupfer DJ (1989) The pittsburgh sleep quality index: A new instrument for psychiatric practice and research. Psychiatry Res 28: 193-213.
- Steffen AM, McKibbin C, Zeiss AM, Gallagher-Thompson D, Bandura A (2002) The revised scale for caregiving self-efficacy: Reliability and validity studies. J Gerontol B Psychol Sci Soc Sci 57: 74-86.
- Bédard M, Molloy DW, Squire L, Dubois S, Lever JA, et al. (2001) The Zarit Burden interview: A new short version and screening version. Gerontologist 41: 652-657.
- Ory MG, Hoffman RR, Yee JL, Tennstedt S, Schulz R (1999) Prevalence and impact of caregiving: A detailed comparison between dementia and nondementia caregivers. Gerontologist 39: 177-185.
- Bentler PM, Bonett DG (1980) Significance tests and goodness of fit in the analysis of covariance structures. Psychological bulletin 88: 588.
- Cohen-Mansfield J, Werner P, Freedman L (1995) Sleep and agitation in agitated nursing home residents: An observational study. Sleep 18: 674-680.
- Volicer L, Hurley AC (2003) Review article: Management of behavioral symptoms in progressive degenerative dementias. The Journals of Gerontology Series A: Biological Sciences and Medical Sciences 58: 837-845.
Author Details
Rohith Nama1*, Tonya Smith-Jackson1, Younho Seong1, Matthew McCullough1, John Lach2, Azziza Bankole3, Martha Anderson4 and Temple Newbold3
1North Carolina A&T State University, USA
2University of Virginia, USA
3Carilion Center for Healthy Aging, USA
4Virginia Tech - Carilion School of Medicine, USA
Corresponding author
Rohith Nama, North Carolina A&T State University, USA.
Accepted: February 14, 2019 | Published Online: February 16, 2019
Citation: Nama R, Smith-Jackson T, Seong Y, McCullough M, Lach J, et al. (2019) Designing Dementia Caregiver Empowerment Interventions through a Simulation: A Conceptualization Study. Int J Healthc Syst Eng 1:001.
Copyright: © 2019 Nama R, et al. This is an open-access article distributed under the terms of the Creative Commons Attribution License, which permits unrestricted use, distribution, and reproduction in any medium, provided the original author and source are credited.
Abstract
Agitation episodes influence the quality of life of both the person with dementia (PWD) and caregivers. Caregiving is challenging, with high workloads and barriers such as fatigue, lack of sleep, and unpredictable episodes of agitation among PWD. Advanced technologies such as cyber-human systems are increasingly considered as means to alleviate some of the stress experienced by caregivers of PWD. To ensure user-centered design of cyber-human systems, it is important to conceptualize the sociotechnical challenges using a cybersociophysical framework and problem space within the context of dementia caregiving, especially as it relates PWD agitation, a major contributor to psychosocial stress. An interdisciplinary collaboration was implemented to use a scaled world, and behavioral and computational simulation as a beginning step to conceptualize the cybersociophysical systems space and the complex interplay between physical space, environmental factors, and the dyad of study (caregiver + PWD). In this research, a simulation study was conducted to test a structural equation model (SEM) that demonstrated the influence of behavioral and environmental factors as well as caregiver disempowerment on agitation propensity. The outcomes of the SEM presented the possibility of developing interventions using simulated data and provided information to deploy a more effective approach in actual residential dwellings. Recommendations for future studies are to obtain frequent caregiver observations, apply a means to prioritize the most important environmental factors, and combine both psychometric measures and behavioral measures to identify antecedents to agitation.
Keywords
Caregiver, Cyber-human System, Dementia, Dementia interventions, Structural equation models
Introduction
Dementia is classified as a neurocognitive disorder [1]. There are many causes of dementia. Alzheimer's is the most common cause of dementia and accounts for 60%-80% of the cases [2,3]. Alzheimer's disease is characterized by a decline in memory and other cognitive capabilities that affects the day-to-day activities of the person with dementia. As of 2015, 46.8 million people worldwide are diagnosed with dementia and the total estimated cost of the disease is $818 million per year [4]. Age is the greatest risk factor for Alzheimer's disease, and it was estimated that 5.1 out of 5.3 million who suffer with dementia in United States are 65 or older [5]. The prevalence of dementia is increasing rapidly, and it was estimated that 131.5 million people around the world will suffer with dementia by 2050 [4]. At present, dementia can neither be cured nor prevented [6]. However, adequate caregiving can have a significant influence on the severity of the disease and may reduce the long-term effects of the disease on persons with dementia. Nevertheless, severity of the agitation episodes results in higher psychological burden and depression levels among caregivers, which in turn affects the quality of care that the PWD receive [7].
Non-pharmacological interventions to prevent agitation episodes can improve the quality of life for PWD and caregivers [8]. However, reliable tools and resources required to develop non-pharmacological interventions are very limited, especially those technologies relying on digital, Internet-of-Things (IoT), wireless networks, and sensors. The technology to predict agitation episodes and present interventions to the caregivers is being developed through a collaborative project between Virginia Tech - Carilion School of Medicine (VTCSOM), North Carolina A&T State University (NCAT) and University of Virginia (UVA). The project is referred to as Behavioral and Environmental Sensing and Intervention for Dementia Caregiver Empowerment (BESI). The goal of BESI is to empower caregivers by designing and developing tools and interventions to prevent or mitigate agitation episodes. Another goal is to engage caregivers in more active and hopefully effective processes in caregiving.
The BESI project focuses on developing a Cybersociophysical System (CSPS), where cyber-physical elements are environmental and wearable sensors and social elements are comprised of caregiver observations, the PWD, and the living environment. Caregivers provide their observations using an android application run on a tablet platform and interface. The overall system includes a tablet for the caregiver, a wrist-worn watch for the both members of the dyad, environmental sensors in the home, and a base station is collectively referred to as the BESI system. The BESI system is being designed to:
• Measure the correlation between various environmental and behavioral factors on agitation episodes using the data obtained from CSPS.
• Develop data models that could establish the relationship between agitation episodes and the factors measured using CSPS.
• Develop and present interventions to caregivers in the form of Just-In-Time (JIT) notifications using the BESI tablet interface application.
The project discussed here is explores data structuring and modeling approaches using behaviorally and computationally simulated data. Lessons learned were transferred to field studies conducted by the BESI team.
The process of developing and presenting interventions in the BESI system is presented in Figure 1. The goal of this research was to lay a foundation for the BESI project through the simulation study and understand the complexities involved in the process of developing interventions. To achieve the preliminary work, a structural equation model was used. This research used both the physical and computer simulation to model inputs for agitation patterns, caregiver attributes, and environmental and behavioral factors. The SEM was developed and tested to examine the effect of various environmental and behavioral factors as well as the influence of cross-cultural patterns of caregiver disempowerment on agitation propensity in PWD.
Background
Frequent agitation episodes increase burden on caregivers thereby influencing the decision to transition loved ones to long-term care facilities [9]. Non-pharmacological interventions that caregivers provide can prevent or minimize the occurrence of agitation in PWD. As the associated neuropsychiatric symptoms of the disease increase, more skilled interventions are necessary [10]. However, caregivers have little knowledge or may have limited awareness of how to interact due to stress of the factors leading to agitation episodes. In addition, cultural attributes among caregivers have an impact on the burden and depression levels experienced. A study comparing 175 African American and White families indicated that White families exhibited increased burden and decreased life satisfaction because of the caregiving process [11]. Moreover, African-American caregivers reported less burden and depressive symptoms when compared with White caregivers [12]. In another study, males exhibited higher self-efficacy and greater satisfaction in the caregiving process [13]. It should be noted that studies on ethnic and cultural differences in dementia caregiving continue to yield mixed results. Therefore, interventions designed to assist caregivers should be designed inclusively by examining the influence of cross-cultural attributes of caregivers on the agitation episodes in PWD.
Methodology
Initially, a simulation study (both physical and computational) was conducted to simulate various environmental and behavioral attributes of PWD, agitation patterns in PWD, and caregiver attributes. Subsequent sections explain the process of data simulation along with hypotheses and SEM that is tested in this research.
Hypotheses
In this research, agitation propensity was operationalized as the combination of Teager energy, CMAI scores, sleeping pattern and depression of PWD. Factors associated with caregiver such as caregiver self-efficacy, depression and burden were the functions of caregiver disempowerment. Similarly, environmental factors were operationalized as the linear combination of temperature, light, and noise. Twelve hypotheses were tested in this research using SEM and are presented in the Table 1.
Structural Equation Model
A SEM was developed based on the hypotheses and is presented in Figure 2. The model uses 12 Manifest and 3 Latent variables as shown in Table 2. The latent variables in the SEM are agitation propensity in PWD, caregiver disempowerment and environmental factors. Three measurement models were the agitation propensity in PWD, caregiver disempowerment, environmental factors and the manifest variables associated with these variables. The structural model was the hypothesized relationship among the latent variables. The goal of the analysis was to test the model and hypothesis to assess the relationship among various latent variables and the manifest variables associated with them.
Data Simulation
Physical simulation
A living space physical simulation as shown in Figure 3 was setup in a laboratory of dimensions 22 ft. × 8 ft. × 11 ft. The living space model had a bedroom, living room, kitchen and a bathroom. Two relay stations (system of sensors) were installed, one in the bedroom and one in the living room. A base station and Wifi were set up in the corner of the living room. Each relay station has a temperature, light, and noise sensor (Table 3), which transmits data continuously to the base station. The data was collected using two student researchers as actors who wore a shimmer device (aka, accelerometer) on the wrist and exhibited physical and verbal agitation symptoms. Student researchers received a task list of regular activities as well as the agitated behaviors they should exhibit through the duration of data collection. Data was collected for six hours for each participant, two hours on each part of the day (i.e. Morning, Afternoon, and Evening).
Teager energy
In this research, accelerometer data was processed using the Teager energy equation to understand the agitation patterns in PWD [14].
Equation 1. E ≅ A2 ω2 [15]
Computer simulation
The data associated with behavioral attributes of PWD and caregiver disempowerment were simulated using the attributes assigned to caregivers and scoring patterns of assessment tools. Agitation in PWD was evaluated using the Cohen-Mansfield Agitation inventory (CMAI) [16], depression was estimated using the Cornell scale for Depression [17], and sleep quality was assessed using Pittsburg sleep quality index [18]. In addition, caregiver self-efficacy was evaluated by the Revised Scale for Caregiving Self-Efficacy [19]. The Zarit Burden Scale-short form was used to assess caregiver burden [20] and depression in caregivers was estimated using Center for Epidemiological Studies Depression Scale Caregivers [21].
Behavioral attributes of PWD
Pittsburg sleep quality index and Cornell scale for depression were used to assess sleeping patterns and depression. Scores for these psychometric indices were identified based on the agitation literature. As this research has two actors, the data was simulated in such a way that both the actors had sleeping disturbances and only one actor exhibited high depression symptoms.
Attributes assigned to caregiver persona: In this research, personas were generated to use for a dual purpose- (1) As a communication and design tool for the interface design of the BESI tablet interface application; (2) For the purpose of data simulation. The scores assigned to personas represent the cultural patterns associated with dementia caregivers as they were generated based on the gender and ethnicity factors as presented in literature review. The scores were simulated and assigned to represent certain factors in each persona to serve the dual purpose (Table 4 and Table 5).
Method
Data was simulated using three different sequential methods until the significant factor loadings and correlation among latent constructs was obtained after testing the SEM. The flow chart shown in Figure 4 demonstrates the procedure followed for data analysis. The data from the integrated sensor network is physically simulated and the data that was supposed to be obtained from the BESI tablet interface application was computer simulated. Later, the data was processed, integrated and analyzed using SEM. Finally, SEM results were validated with prior research findings and a foundation to develop interventions was presented.
Results
The primary objective of the research was to determine whether disparate data types could be structured and modeled using SEM in the context of agitation and a living space. Secondary to this goal was to examine whether data and models could be developed to explore the influence of cross-cultural patterns of caregivers on agitation propensity in PWD in a simulated living space. In this research, the simulated data for two actors and two caregiver personas was available. The initial plan was to integrate the data of each persona with the actor data and observe the variation in the influence of caregiver disempowerment on agitation propensity in PWD (as presented in Figure 5). However, due to the limitations of the computer simulated data, there was no possibility to test four different combinations and examine the influence of cross-cultural patterns in the current research study. Therefore, one set of computer simulated data was integrated with the physical simulation data for two actors and tested with SEM. The following sections demonstrate the results of the data analysis.
In this research, the Normed Fit Index (NFI), Non-Normed fit index (NNFI) and Confirmatory Fit Index (CFI) were used primarily to assess the reliability of the estimates of SEM [22]. The values of NFI, NNFI, and CFI fall between '0' to '1' and the value close to '1' suggests a good fit, which means a value greater than 0.95 indicates a very good fit and a value close to 0.9 and 0.95 indicates adequate fit. However, chi-square/df and Root Mean Square Error of Approximation (RMSEA) values are presented. The rationale for using these fit indices is related to the nature of data, which contained large, disparate data sets. All of the variables used exhibited non-normal distributions as well. The cut off for p-value 0.01 was selected for this research.
Data simulation method 1
Initially, the data was generated randomly by ignoring the correlation among the variables. Table 6 presents the results of the confirmatory analysis of data simulation method 1. The results demonstrate the unreliability of the data simulated. The reason for not obtaining any significant values for the computer-simulated data was the method adapted for simulation, which used the syntax from a random number generator. These random values did not exhibit any patterns; thus, affecting the covariance between each manifest as well as the latent variable. A further attempt was to simulate the data in such a way that a covariance pattern existed among the manifest variables that are associated with each latent variable in the SEM using the aforementioned literature-based numerical inputs and distributions.
Data simulation method 2
For method 2, the data was generated by inducing the correlation among manifest variables. Unlike the results of the data simulation method 1, the values associated with fit indices and factor loadings for manifest variables were significant. However, there was no correlation between any two latent variables (Table 7), which is one of the primary interests of this research study. The reason for the failure to obtain the expected results was the method used to simulate the data for caregiver self-efficacy. Only one range of values (either > 70 or < 20) with limited data were used for the self-efficacy scale, which lacked variation in revealing patterns to correlate with the values associated with agitation propensity. This demonstrates a key basic finding that in order to find the correlation between any variables, there is a need to have enough data points to exhibit various patterns; thus, in field studies, it is important to ensure instruments provide a range of values or values are adjusted based on psychometric re-scaling. To understand the correlation among latent variables and a way to develop individualized interventions, the data was further simulated by categorizing each manifest variable into three different sets and further analysis was conducted.
Data simulation method 3
As the data simulated in this method led to some insights, the data is tested with two actors as presented in Figure 5.
Measurement Model results for actor 1
The results in Table 8, Table 9 and Table 10 present the final estimates of the modified measurement model. The CFI, NFI, and RMSEA values present that the model is a good fit. Initially, the Teager energy variable had an estimate of 0.006 so it was dropped from the model. In addition, the standard error for temperature variable was significantly low and hence dropped from the model. Therefore, the estimates of other factor loadings, fit indices, and correlation estimates were slightly improved. All estimates were significant and present acceptable factor loading values. The values obtained for the correlation among the latent factors were significant. Altogether, these findings support adequate reliability and validity of the model of the constructs and their indicators. Therefore, further path analysis was conducted to understand the structural model.
Structural model results for actor 2
The theoretical model tested was constructed based on the results of confirmatory factor analysis. As mentioned Teager energy and temperature, variables were dropped from the model. Further, higher factor loadings for each latent variable were assigned a value of '1'. The results of the path analysis for the structural model are presented in Table 11 and Table 12. The fit indices demonstrate good fit and the path coefficients between latent constructs were significant, thus demonstrating the reliability of the SEM presented in Figure 2.
Measurement model for actor 2
These results presented in Table 13, Table 14 and Table 15 were the findings of the measurement model. Similar to the results of the actor 1 data, Teager energy exhibited a poor factor loading; thus, deleted from the model. The NNFI value was very close to 0.9 and hence considered acceptable. Chi-square/df value was not satisfactory, but its significance in this research was ignored because of its sensitiveness to sample size. All factor loadings of manifest variables were significant and represented acceptable estimate values.
Structural model results for actor 2
Fit indices and factor loadings demonstrated that findings of the structural model showed good reliability and validity. Except for chi-square/df, the other fit indices indicated the validity of model and factor loadings of all the manifest variables were significant. The path coefficient value between agitation propensity in PWD and caregiver disempowerment was 0.99, which may not be the case in real time. Overall, the model demonstrated a good fit and presented the covariance and correlation factors associated among various latent variables and their corresponding manifest variables (Table 16 and Table 17).
Discussion
The purpose of this research was to conduct a simulation study to obtain the data, structure the data, and then test an SEM to understand the triggers of agitation episodes in PWD. The initial plan was to observe the influence of cross-cultural attributes of caregivers on agitation propensity in PWD by testing the different combinations of data as shown in Figure 4. However, lack of various patterns within the computer simulated data restricted the scope of this research, thus leaving no possibility to observe the cross-cultural patterns develop inclusive interventions. Nevertheless, the SEM developed in this research demonstrated the possibility of developing individualized interventions. The SEM presented in Figure 2 was tested using the combination of data obtained from all the three methods used for computer simulation along with the physical simulation data. The findings of the first two methods were not reliable as the results demonstrated the lack of significant factor loadings for manifest variables and correlation among latent variables. However, based on the information learned from the first two methods, data was simulated in such a way that it exhibited different patterns in a single day. The results in both cases (for actor 1 & actor 2) demonstrated that model provides a good fit and presented the significant correlation between latent variables such as environmental and behavioral factors, caregiver disempowerment and agitation propensity in PWD. Figure 6 and Figure 7 demonstrate the results of data analysis for both the actors.
Figure 6 and Figure 7 present the correlation between various environmental and behavioral factors along with caregiver attributes on agitation episodes for both the actors. Teager energy variable was dropped from the model for both the actors because of the low estimate of factor loading value. Lack of balance between the patterns associated with the simulated data and Teager energy value was the primary reason for the low estimate. Availability of large data set that exhibits various patterns for all the variables can result in acceptable factor loading value for Teager energy variable as demonstrated in the pilot study [14]. However, CMAI scores that are used to validate agitation episodes obtained a good factor loading value and can be used along with the Teager energy values, which are the primary interest of this research [16]. Based on these findings, in order for the results of the BESI research study to be accurate and reliable, a suggestion is to use a combination of Teager energy scores and CMAI scores to detect agitation patterns in PWD. As the Teager energy data set was large (data were collected per second), another recommendation is to collect the assessment score data more frequently so that variations in the characteristics measured would correlate with the Teager energy scores. The estimates of CMAI, sleeping patterns, and depression scores in two different data sets of method 3 were close to 0.8 and 0.65, respectively (Figure 6 and Figure 7). The positive correlation shown in this research is consistent with prior research findings [23,24]. However, the strong correlation in this model may not be similar with real time data but the effect should be consistent with present and prior findings.
One of the primary focuses of this research was to find the influence of environmental variables on agitation propensity. The simulation study findings showed that the environmental factors could be harnessed and structured to model an impact on` agitation propensity with estimates of 0.21 and -0.3 in two different data sets (Figure 6 and Figure 7). If the simulated process transfers to the residential setting, then these findings hold the key to developing individualized interventions related to the environmental factors. Findings of the path coefficients for caregiver disempowerment and agitation propensity in PWD were 0.85 and 0.99 for actors 1 and 2, respectively. These positive path coefficients are consistent with the literature [9]; however, the strength might be lower with real-time data. These findings demonstrate that agitation propensity and caregiver disempowerment, in terms of the “behavior” of the data, are directly proportional to each other. These findings suggest that decreasing the agitation propensity by introducing interventions would help to provide useful information to caregivers to effect caregiver efficiency. Further investigation is necessary to recommend precise interventions for the caregiver. For example, a possible intervention for actor 2 is to alter the environmental factors to prevent agitation episodes; however, there was no specific information available about ways to alter- such as increase/decrease the temperature, light, or noise to a certain level. In order to be able to present precise interventions, a larger data set is needed with data collected in real time environments of PWD. Another recommendation is to focus on a way to simplify the information provided to the caregiver and present accurate interventions.
Limitations
This research used behaviorally simulated data, which may not be equivalent to real-time data. The attributes assigned to personas and the characteristics of PWD may vary from context to context, which demonstrates the need for a real-time study. Further, the computer simulated data in this research exhibits limited patterns, which might have influenced the outcomes of this research.
Conclusion
In this research, simulation and SEM were used to inform further activities in the larger, human-subjects based BESI field studies. The outcomes of the simulation study demonstrated the possibility to develop interventions using the SEM by examining the influence of various environmental and behavioral factors as well as caregiver disempowerment on the agitation propensity in PWD. However, further research with large real-time data sets on actual human subjects is required to be able to develop and present interventions. Currently, field studies are being conducted with human subjects. When the interventions are developed using the data obtained from the real-time deployment of CSPS, they could provide outputs for recommendations and user requirements to decrease the burden of caregiving, which in turn can decrease the probability of relocating PWD to long-term care facilities. These interventions may also serve as a resource to healthcare providers for treatment decisions, accordingly, thus enabling the possibility of effective caregiver-healthcare provider partnerships. As simulated data can differ significantly from the data obtained through the real-time deployment of technology, a recommendation is to validate the model (shown in Figure 4) with real-time data. Based on the observations and learnings from the research study, a list of recommendations was developed to assist in future studies regarding the BESI project.
1. Monitor the PWD and environment continuously using CSPS for a long time to be able to develop precise interventions.
2. Use a combination of Teager energy and CMAI scores to validate agitation patterns.
3. Devise a way to obtain frequent observations from the caregiver regarding behavioral attributes of PWD, such that patterns can be correlated with Teager energy.
4. Do not transform the data, if data exhibit a non-parametric distribution, use the asymptotic distribution free methods such as Weighted Least Square to perform SEM.
5. Develop interventions by observing various attributes of caregivers such as self-efficacy, burden, and depression.
6. Devise a way to obtain notifications when the data is not being transmitted to the base station for a significant amount of time.
Acknowledgements
The National Science Foundation and National Institutes of Health (Award- IIS-1418622) supported this work. Authors would like to acknowledge Sriram Raju Dandu (UVA) and Abhimanyu Salin for their help with the data processing. Further, thanks to Daran Wynn and Harshitha Meda for their support in data collection. Finally, we would like to extend our appreciation to all the collaborative researchers from NCAT, UVA, and VTCSOM.