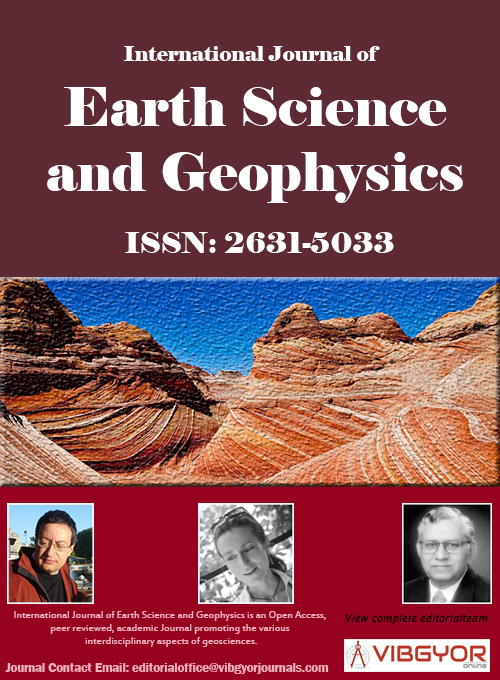
International Journal of Earth Science and Geophysics
(ISSN: 2631-5033)
Volume 7, Issue 2
Research Article
DOI: 10.35840/2631-5033/1848
Computational Cut-Off Grade Modeling: A Review
Sandeep Prasad1* and Ajay Kumar2
Table of Content
Figures

Figure 1: Adopted flow chart of computational....
Adopted flow chart of computational cut-off grade modeling a review.
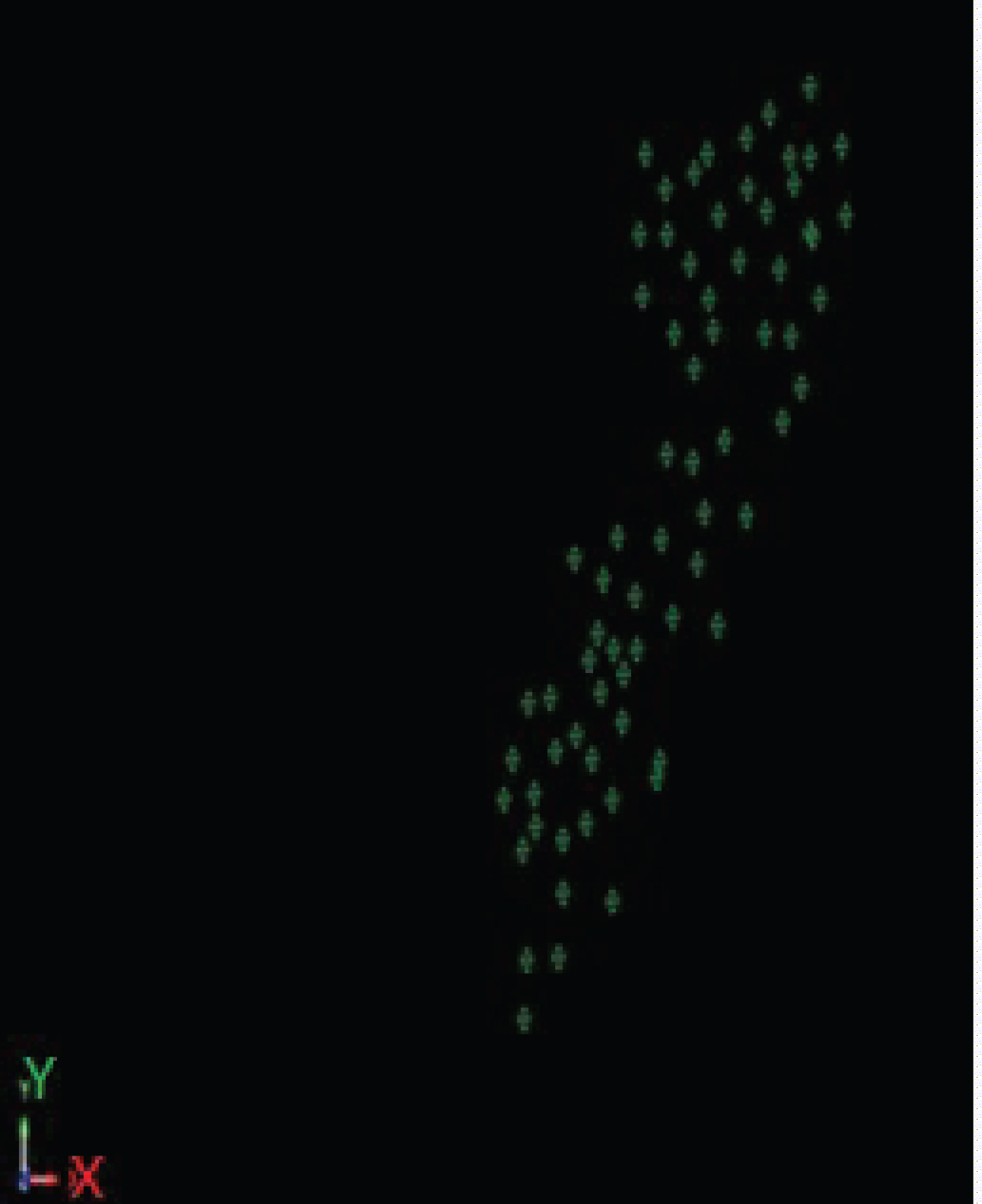
Figure 2: Step first positioning of X-axis....
Step first positioning of X-axis, Y-axis distribution of borehole values (Using Table 1) in model and computed display in the monitor.

Figure 3: Steps second development of section....
Steps second development of section (i.e., using steps of borehole values to intersection connection) to identify grade level in the model.

Figure 4: Steps thirds represented of surface....
Steps thirds represented of surface grade in model.

Figure 8: Statistical analysis ore grade...
Statistical analysis ore grade: Several samples versus the class.
Tables
Table 2: Report of borehole section by a solid body.
Table 3: Creation of a geological database (assay value).
Table 4: Grade estimated.
References
- Lasky SG (1950) How tonnage and grade relations help predict ore reserves. Eng Min J 151: 81-85.
- Annels AE (2012) Mineral deposit evaluation: A practical approach. Springer Science & Business Media.
- Henley S (2000) Resources, reserves and reality. Earth Sci Comput Appl 16: 1-2.
- Galloway WE, Hobday DK (2012) Terrigenous clastic depositional systems: applications to fossil fuel and groundwater resources. Springer Science & Business Media.
- Dai B, Wang L, Jia M, Liu H, Jing Y, et al. (2007) Application of 3D digital modeling in a copper mine [J]. Geol Prospect 3.
- Taylor HK (1985) Cut-off grades-some further reflections. Inst Min Metall Trans Sect A Min Ind 94.
- Verly G, David M, Journel AG, Marechal A (2013) Geostatistics for natural resources characterization. Springer Science & Business Media, 122.
- Curi A, Pereira MA, de Sousa WT, Silva VC (2013) Final open pit design for Monte Raso phosphate mine. International Journal of Modern Engineering Research 3: 3780-3785.
- Ahmadi MR (2018) Cut-off grade optimization based on maximizing net present value using a computer model. J Sustain Min 17: 68-75.
- Lane KF (1988) The economic definition of ore: Cut-off grades in theory and practice. Mining Journal Books, London.
- Liimatainen J (1998) Valuation model and equivalence factors for base metal ores. Proceeding mine Plan Equip Sel 317-322.
- Zhang S (1998) Multi-metal recoverable reserve estimation and its impact on the Cove ultimate pit design. Mining Engineering 79-79.
- Baird BK, Satchwell PC (2001) Application of economic parameters and cut-offs during and after pit optimization. Mining Engineering 33-40.
- Malewski J, Krzeminska M (2012) Dependence of mine revenue on the grade of copper concentrate. Physicochem Probl Miner Process 48: 545-554.
- Ataei M, Osanloo M (2003) Determination of optimum cut-off grades of multiple metal deposits by using the Golden Section search method. J South African Inst Min Metall 103: 493-499.
- Osanloo M, Ataei M (2003) Using equivalent grade factors to find the optimum cut-off grades of multiple metal deposits. Miner Eng 16: 771-776.
- Lerchs H (1965) Optimum design of open-pit mines. Trans CIM 68: 17-24.
- Zhao Y (1992) A new optimal pit limit design algorithm. Proc 23rd APCOM 423-434.
- Whittle D, Vassiliev P (1998) Synthesis of stochastic recovery prediction and Cut-off optimization. In: Mine to Mill Conference 53-55.
- Shinkuma T (1998) The Cut-off grade and the rationality of ‘The Rule of Thumb of Mines. Stud Reg Sci 28: 55-66.
- Ajay Kumar VS, Kumar L, Vardhan A, Kumar R (2014) A GIS based application and analysis features of land survey of urban area of raster data map. Int Conf Adv Eng Technol.
- Kumar A, Gorai AK (2018) Geo-spatial estimation and forecasting of LULC vulnerability assessment of mining activity: A case study of Jharia coal field, India. J Remote Sens GIS 7: 1-16.
- Gai K, Qiu M (2018) Optimal resource allocation using reinforcement learning for IoT content-centric services. Appl Soft Comput 70: 12-21.
- Gai K, Qiu M (2018) Reinforcement learning-based content-centric services in mobile sensing. IEEE Netw 32: 34-39.
- Gai K, Lu Z, Qiu M, Zhu L (2019) Toward smart treatment management for personalized healthcare. IEEE Netw 33: 30-36.
- Kumar A, Villuri VGK (2015) Role of mining radar in mine slope stability monitoring at open cast mines. Procedia Earth Planet Sci 11: 76-83.
- Kumar A, Rathee R (2017) Monitoring and evaluating of slope stability for setting out of critical limit at slope stability radar. Int J Geo-Engineering 8: 1-16.
- Staples M (1995) Whittle the muppets. In: Proceedings of Optimizing with Whittle Conference, 135-142.
- Verly G (2005) Grade control classification of ore and waste: a critical review of estimation and simulation-based procedures. Math Geol 37: 451-475.
- Diehl P, David M (1982) Classification of ore reserves/resources based on geostatistical methods. CIM Bull 75: 127-136.
- Dowd PA, Xu C (1999) The financial evaluation of polymetallic mining projects. In: K Dagdalen, Proceedings of the 28th International Symposium on Application of Computers and Mathematics in the Mineral Industries.
- Cetin E, Dowd PA (2016) Multiple cut-off grade optimization by genetic algorithms and comparison with grid search method and dynamic programming. J South African Inst Min Metall 116: 681-688.
- Shinkuma T, Nishiyama T (2000) The grade selection rule of the metal mines; an empirical study on copper mines. Resour Policy 26: 31-38.
- Goodwin LD (1996) Determining cut-off scores. Res Nurs Health 19: 249-256.
- Naik HK, Das P (2018) Optimization study of a surface mine and grade monitoring using SURPAC. 1-7.
- Wharton CL (1996) What they don’t teach you in mining school--tips and tricks with pit optimizers. Surf Min 17-22.
- Rardin RL, Rardin RL (1998) Optimization in operations research. Prentice Hall, Upper Saddle River, NJ.
- Wheeler AJ, Rodrigues RL (2002) Cutoff-grade analysis at Fazenda Brasileiro: Mine planning for declining gold prices. Min Technol 111: 35-46.
- Nalder IA, Wein RW (1998) Spatial interpolation of climatic normals: Test of a new method in the Canadian boreal forest. Agric For Meteorol 92: 211-225.
Author Details
Sandeep Prasad1* and Ajay Kumar2
1Department of Mining Engineering, AKS University, Satna, India
2Department of Mining Engineering, NIT, Rourkela, India
Corresponding author
Sandeep Prasad, Department of Mining Engineering, AKS University, Satna, 485001, India
Accepted: August 03, 2021 | Published Online: August 05, 2021
Citation: Prasad S, Kumar A (2021) Computational Cut-Off Grade Modeling: A Review. Int J Earth Sci Geophys 7:048
Copyright: © 2021 Prasad S, et al. This is an open-access article distributed under the terms of the Creative Commons Attribution License, which permits unrestricted use, distribution, and reproduction in any medium, provided the original author and source are credited.
Abstract
An entry into the mining profession is associated with many sectors that affect the environment, economy, and livelihood. Here, we have concentrated on the ore grade obtained from the extraction of the mining region. These ore-grade materials have limited on the mining region. Still, demand increases day by day-also affects the ore-grade level of materials as the supply situation. So, we have reviewed computational-based research on cut-off grade modeling techniques. We have to know the grade reserver boundary range defined levels of obtained grade qualities. Eventually, the conventional method has been done complex, and this worked very tediously. Many experts have done soft computing techniques over cut-off grade modeling using ordinary kriging, nearest neighbor, and inverse distance. Here, we have reviewed these techniques on three-parameter values like assay, collar, and grade value on the leading role for cut-off grade modeling. Also, the estimated reserved is represented as a graphical for open-pit models like borehole, section model, surface model, block model, etc., and computed results. Therefore, this review paper has expressed an interest in cut-off grade monitoring and emphasized advanced performance-based soft computing techniques for the future perspective.
Keywords
Soft computing, Cut-off grade, Open-pit model, Mining ore
Introduction
Ore is the heart of mining land-an occurrence of reserved quality grade categorized with a high level, low level, and waste. An underground material has dug by mining operation to extract materials obtained at the surface, a challenging task instead for ore-grade. The overall scenarios of ore finding have first the reconnaissance survey, which is an objective task for ore terrain an initialization and planning. Types of grade quality have demanded market values and tremendous growth, and a fast increasing demand for ore productions. Ore deposits can be categorized into three types massive deposits, vein deposits, lode type deposits. Also, it is horizontally stratified reserves of sedimentary origin with a thin or thick covering of overburden [1]. Mine planning plays a vital role in total ore reserves and the average grade of the deposit [2]. Existing methods are ore mining, and cut-off grade estimation in single ore deposited classified through geometric or geostatistical [3]. Cut-off grade is discriminated between waste and ore present over open cast mines [4]. In situ of open-pit mines is classified through present grade obtained versus continued operation to end level be examined. It is complex decisions about the grade types for qualitative of cut-off grade level [5]. Both merit and demerit are essential roles in mining ore for direct or after processing of ore sale, and this extraction of ore is accumulated with waste. It is a collection of waste dumps for the treatment of dumps waste in the plant processing for sale [6]. In Chuquicamata open pit mine early 1960's, an optimization technique is used to evaluate cut-off grade level and enhance net present value (NPV). In Newmont gold corporation's early 1980s, the large scale linear programming model is significant the scheduling of mine and mill production for process costs saving [7]. Monte Raso phosphate mine, Brazil is designed for ultimate pit limits by the drill hole information and the topographic map [8]. According to the five-year plan of Gol Gohar iron mine, No.1 with the computer model reference of NPV data is the mines life correlated with grade tonnage. The change in optimum cut-off grade and NPV like 48.65% and 18582 billion rails, respectively [9]. Similarly, deposit and time cost management is most useful in a multi-criteria decision model for grade ratio and setting an uncertain price plan production [10-12]. Also, the evaluation of ore mining grades for some types of methods is most popular, likes critical level, net smelter revenue (NSR), single grade cut-off approach, dollar value cut-off approach [13-16]. The ore production is a valuable strength for estimating cost and commodity revenue that is checked by the economic block value and the break-even based. Further, a level of the cut-off grade will be decided, which will be profitable or not [17,18]. The scheduling has used mine pit development level by day/month/yearly based production. Also, the perspective of ore estimation or ongoing is very easily of production calculated by scheduling [19]. The scheduling process is obtained from best ore, waste ore, and the operating mines updated from time to time at a particular point of ore extraction location [20]. But, grade monitoring is a significant effect on most variable factors for operation in ore planning. Elsewhere, remote sensing technology development is a growing change in the mining industry, automation of machinery, and fast computation [21,22]. The machine learning scenario is vital in ore resources intimation, a type of reinforcement learning with the internet of things (IoT) for ore resource service of data transformation from field to working station [23]. Also, it is applied for data transfer, a more comfortable aspect of hotspot without arrangement sending of data [24]. Healthcare and safety are significant challenges for mines operation, but these are negotiable issues for a worker. It is required only skilled workers for the work operation [25]. The mining land is some factors like surface stability, rainy weather, etc. that is a problem attracted into time for geological survey [26,27].
Prospective of work
This paper has been sighted a software capability in reserve determination, which can be proved beneficial in real-time mine planning. In the soft computing model, the mining industry is deployed very quickly to evaluate various parameters such as grade estimation, reserve estimation, the life of the mine, short and long-term mine planning, etc. [28]. Modeling is beneficial for top management people that resources and analyses can easily. So, these steps can be taken to ensure steady production.
The geological terrain is iron ore borehole information that is obtained from the geological survey of India. The soft computing model is a very catchy representation of the ore deposit map and estimates the grade value's reserver. This estimation result is applied comparatively by techniques, i.e., inverse distance, nearest neighbor, and ordinary kriging. The estimation technique is more effective than the fulfillment of aspects of optimization of grade level, map design, and future planning of ore deposits.
Methods and Materials
The following methodology flow chart has been adopted to fulfill the objective criteria for modeling shown in Figure 1.
Geological impacts and Soft computing
The current practice of ore extraction begins with geological conditions and information to be related to such an ore area that it is ores or not. The raw data is collected from a field survey: Assay data, grade data, coordinate location, and map plan. The geological block modeling is used to determine each block's grade through different grade estimation techniques [29]. Mine planning is a multi-parameter optimization problem, where more than one parameter is involved in mine production [30]. These parameters are interrelated, where any change in one parameter affects all other related parameters [31]. Therefore, the incorrect information value of one parameter determines the following parameter is not possible. Mine life is decided by using all the resources present in the ultimate pit limit design. Ore grade is a concentration of grade level for interest in a potentially mineable ore deposit. Before the cut-off grade is decided, the ore body's average grade level is calculated from the reserve estimated through modeling techniques [32]. Finally, an ultimate pit is designed so that maximum ore is extracted within the minimum period. Also, these should be done by abiding by proper legislation norms. The soft computing model helps the mining industry perform the design of pushbacks, ultimate pit limit analysis, and determine yearly mine plans. Pushback generation is part of the planning and scheduling process. It is evolved to surface in the intermediate pits leading to ultimate pit limits. Opencast mine planning is conducted by first generating a representative model of the ore body. Eventually, it is divided into several blocks and sub-blocks known as block modeling, in which slice plans are developed [33]. While designing the pit, it should be kept in mind that the stripping ratio should be economical to minimize losses [34].
Evaluation of Stepwise Step Stages
Implementation of borehole
The use of soft computing is derived form of excel file data likes assay, survey, collar, geology. It is extracted from borehole data as shown in Table 1 [35]. Further, it is explored borehole or drill hole information using soft computing model that data is inputted in the software, the drill holes can be viewed in Figure 2.
This borehole is displayed, the geology patterns and the lithology distinguished by using collar variants. It is discriminated against by the mineral concentrations by up and down with different colors. After that, it is displayed for the surface condition and estimated the volume of ore. Also, it is composting information of ore reserver encountered by boreholes data. After that, the borehole values are deployed for cut-off grade estimation. A stepwise development has the boreholes through repeated sectioning and digitizing.
Further, the borehole is used for several sections, and all the sections are joined together using a triangulated method. The determination of the ore body is very dependent on the user of the software. Also, it's decided by the amount of dilution and the extent of the pit [12]. The sectioning is generated using a row of boreholes digitizing that determined the ore volume and exempted some boreholes. Some boreholes are the mineral's grade, which will give profit, is significantly less or absent. Thus, it is increased the cost of extraction, adding to the overall cost of mining. After sectioning a row of the borehole, the section is saved that the next row's sectioning is carried out. It is segmented by the generalization of the sectioning and digitizing of the boreholes. The use of triangulation is represented as a node in the shape of a triangular manner. It uses borehole details to triangulate a complete form to interpret an ore body by connecting total borehole points. This orebody is developed by assay data that show a white line in shown Figure 3.
The string file is generated from the collar data excel file, which consists of x- easting, y- northing, z- reduced level. Also, it is developed the digital triangulated model (DTM) by string. It is used for displaying the elevation and surface conditions of the ore area by the boreholes value [36]. Thus, it is a rough image of the surface to be encountered when mining is completed. The DTM is generated by contouring a piece of information for the elevation intervals on the surface. The surface model is developed from DTM by shown in Figure 4. Now, the ore body is joined the segments and the inside of the hollow segment by triangulated. Triangulation is a more precised result, a closed segment otherwise, more error in the open segment. This process is compiled a generated model of the solid ore body using a 2-D or 3-D grid system.
This layer extends the ore body lies min Z value 978m and max Z value 1260m. The report is delineating the area covered and the volume of solid ore body shown in Table 2 and Figure 5.
Development of orebody
The orebody is approached for the development of solid modeling. In the above section, solid modeling is completed computational. But ore body is not covering every drill hole and depth of the complete stand. So, the database's implantation is used to the drill hole and intersected at the end level of ore. Thus, a new ore zone is generated only borehole ids marked for ore, which is crossed the ore body shown in Table 3. A block model is used to care for the influence of the surrounding boreholes to an unknown point. Specifically, it is helped in the grade and reserve estimation of the orebody. It is embedded in vital information for decision-making. Also, it is advised that block size should be around 1/3rd to 1/4th of the borehole spacing. The approached model has used a borehole spacing of 100m and dimension depending on the ore body's layer extent. Eventually, the block size is used in meters as Y-30, X-30, and Z-20. The dimension of Z is used for the height of the bench.
Result and Discussion
Slice plan and pit design
The Slice refers to cutting the ore horizontally at a definite interval. String files are generated containing slice plans of each reduced level. Slice plans are developed during pit designing, where the slice plane of the bottom of the ore body. Slices are represented in different colors depending on the grades. The ore body's slices plan is limestone ore and the significant mineral iron, as shown in Figure 6. The attributes that have followed the calculation for pit designing included the parameters like bench height (10 m), bench slope angle (65°), overall slope angle (46.58°), and berm width (5 m) [32]. Pit design is started from the lower Slice and continuing upwards. Digitizing is done along with the slices for each Slice, and then another slice imported. Also, the generated map is shown in Figure 7. In the pit formation, the volume of the ore inside is calculated by the ore body.
Cut-off grade analysis
The optimum cut-off grade method uses some conditions to eliminate the optimum point of unimodal functions, suggested the uncertainty space, chooses the test point in uncertainty space, and evaluates and compares with the cut-off grade. This process is continually repeated until it reduces the uncertainty spaces and within uncertainty interval (a small specified positive value e). It is used to determine the cut-off grade [37]. Ordinary Kriging methods (OKM) deal with an unobserved location of variables 'Z' based on the weighted average of adjacent observed sites within a given area [38]. An inverse distance method (IDM) is deterministic, like multivariate interpolation with a known grade value. The assigned values to unknown points are calculated with a weighted average of the known points' values. This equation (1) and equation (2) are the mathematical expressions of IDM ([39]).
Where the inverse distance estimate at the estimation location is . Sample points within the search neighborhood are The weights assigned to each sample point is . Euclidian distance between estimation location and sample points is . The power or distance exponent value is p. The expression of equation (3) is a condition of OKM. Once the ore body's estimation has finished, the ore body's report has been generated, which shows the volume, tonnage, average grade of the minerals of the ore body shown in Table 4.
Statistical analysis
Cut-off grade modeling adopted that the steps of the above sections have occurred results. These are generated model outcome maps like the surface model, solid model, and the borehole and block model section. Also, it is generated a report of modeling information that is the aim of determination. A string file is a developed model that the block model depends on borehole data. It is a finalized designed compositing of the block model.
Similarly, the using variogram model is the second kind of cut-off grade modeling that the block model is represented statistical information of a grade shown in Figure 8 [35]. The use of the composite file is the estimation of grade in the variogram model. So, it is required to create new attribute fields and storing the grade value in that field. This estimation method is known as the Similarly; the using variogram model is the second kind of cut-off grade modeling that the block model represents statistical information of a grade shown in Figure 8 [35]. The use of the composite file is the estimation of grade in the variogram model. So, it is required to create new attribute fields and storing the grade value in that field. This method of estimation is known as the ordinary kriging method. Thus, the block model is estimated different constraints as per our choice can be viewed and inputted, and the output image in Figure 9 [35].
The ore body is simulated to mine blocks graphically. The target volume and the target tonnage are pre-set by the user for mining blocks graphically. A selection of the volume of 6499.9 sets up the experimental and tonnage of 34189.9 histograms represented a cumulative as shown Figure 10. The grade attributes histogram describes both the total value and selection value, which is 61.8, as shown in Figure 11 [35].
Conclusion
We have suggested mining for cut-off grade modeling using a soft computation model with a very fast estimation and stochastic framework model obtained as near realistic results. The parameters are used physical, geological information like assay value, grade values, coordinates, and map plan. The use of steps for grade level is open cast mine planning and design of the open pits that it is essential for simply a grade used to distinguish between ore and waste. Also, many grade-level optimization techniques have been successfully applied to resolve the problem of determining an optimal grade-level production schedule. Also, reserve estimation efficiency is compared nearest neighbor and inverse square distance method with the ordinary kriging method. An estimation result shows that both the nearest neighbor method and inverse distance method overestimated the iron percentage. Pit designing is done after perfectly considering all the parameters such as the overall slope angle, bench angle, berm width, and bench height to get the ultimate pit limit. However, the design is done keeping in mind that the pit's boundary does not cross the lease boundary of the mine throughout the process. After the completion of pit design, the amount of ore lost due to part of ore falling outside the pit and its effect on the ore body's grade is calculated. The mining area's perspective is used conventional methods for cut-off grade a very significant effect a parameter in operation and production of mines but compared to the computing model is less effective. Also, the economic and operational viability is significantly less dependent on safety with careful planning and management. In many issues, it is directly affected in cut-off grades, increasing mining costs, and environmental. The operation and management of a large open cast mine having a life of several years is an enormous and complex task.
Acknowledgments
The author would like to thanks, Department of Mining Engineering, AKS University Satna, MP, and NIT Rourkela, Odisha, India.
Financial Funding
No.
Conflict of Interest
The authors declare that they have no conflict of interest.