Predicting Total Number of Deaths using COVID-19 World Data: Application of Linear Regression Model
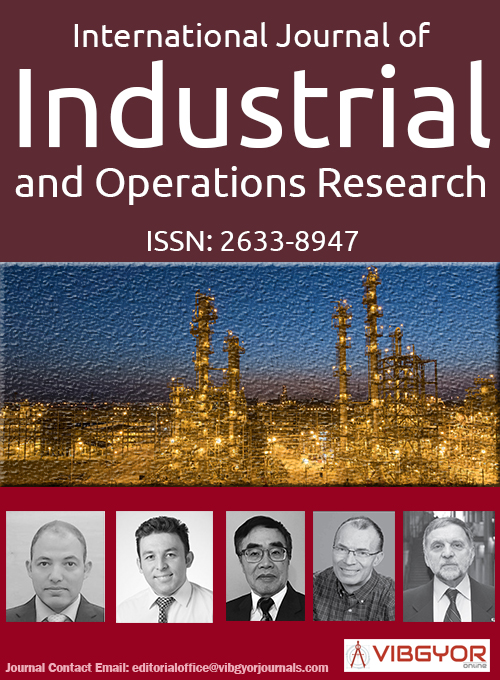
International Journal of Industrial and Operations Research
(ISSN: 2633-8947)
Volume 3, Issue 2
Research Article
DOI: 10.35840/2633-8947/6508
Predicting Total Number of Deaths using COVID-19 World Data: Application of Linear Regression Model
Micaela Siegrist and BM Golam Kibria*
Table of Content
Figures
Tables
Table 1: Descriptive statistics.
Table 2: Regression analysis of the COVID-19 data.
Table 3: Regression analysis on transformed variable.
Table 4: Regression analysis on transformed variable.
Table 5: Regression analysis on transformed variable including x7.
Table 6: Original and predicted values for the last 24 countries.
References
- Francesco Di Gennaro, Damiano Pizzol, Claudia Marotta, Mario Antunes, Vincenzo Racalbuto, et al. (2020) Coronavirus diseases (COVID-19) current status and future perspectives: A narrative review. Int J Environ Res Public Health 17: 2690.
- Hannah Ritchie, Esteban Ortiz-Ospina, Diana Beltekian, Edouard Mathieu, Joe Hasell, et al. (2020) Coronavirus pandemic (COVID-19) - statistics and research. Our World in Data.
- Ghosal S, Sengupta S, Majumder M, Sinha B (2020) Linear regression analysis to predict the number of deaths in India due to SARS-CoV-2 at 6 weeks from day 0 (100 cases-March 14th, 2020). Diabetes & Metabolic Syndrome: Clinical Research & Reviews 12: 311-315.
- Lei Qin, Qiang Sun, Yidan Wang, Ke-Fei Wu, Mingchih Chen, et al. (2020) Prediction of the number of new cases of 2019 novel coronavirus (COVID-19) using a social media search index. Int J Environ Res Public Health 17: 2365.
- Motulsky HJ, Christopoulos A (2003) Fitting models to biological data using linear and nonlinear regression. A practical guide to curve fitting. GraphPad Software Inc., San Diego CA.
- Douglas C Montgomery, Elizabeth A Peck, G Geoffrey Vining (2013) Introduction to linear regression analysis. Wiley-Blackwell.
- James G, Witten D, Hastic T, Tibshirani R (2013) An introduction to statistical learning. Springer, New York.
- Guzman CI, Kibria BMG (2019) Developing multiple linear regression models for the number of citations: A case study of Florida International University professors. International Journal of Statistics and Reliability Engineering 6: 75-81.
- Saleh AK Md E, Arashi M, Kibria BMG (2019) Theory of ridge regression estimation with applications. Wiley, New York.
- Grundy EJ, Suddek T, Filippidis FT, Majeed A, Coronini SC (2020) Smoking, SARS-COV-2 and COVID-19: A review of reviews considering implications for public health policy and practice. Tobacco Induced Disease 18: 58.
Author Details
Micaela Siegrist and BM Golam Kibria*
Department of Mathematics and Statistics, Florida International University, Miami, USA
Corresponding author
BM Golam Kibria, Department of Mathematics and Statistics, Florida International University, Miami, FL 33199, USA.
Accepted: November 04, 2020 | Published Online: November 06, 2020
Citation: Siegrist M, Kibria BMG (2020) Predicting Total Number of Deaths using COVID-19 World Data: Application of Linear Regression Model. Int J Ind Operations Res 3:008.
Copyright: © 2020 Siegrist M, et al. This is an open-access article distributed under the terms of the Creative Commons Attribution License, which permits unrestricted use, distribution, and reproduction in any medium, provided the original author and source are credited.
Abstract
Coronavirus Disease 19 (COVID-19) is a new deadly disease which made its appearance at the end of 2019 in China and it quickly spread worldwide. This paper analyzes which regressors influence the deaths caused by this disease. The variables that were considered were total deaths per million, population density, median age, people age 70 and older, GDP per capita, CVD death rate, diabetes prevalence, smokers- tobacco prevalence, hospital beds per 100,000 people, and total cases per million. After fitting three multiple linear regression models, we found that the variables that are significant when analyzing the deaths by COVID-19 are median age, people 70 and older, tobacco prevalence, hospital beds per 100k people, and total cases of COVID-19 per million.
Keyword
Covid-19, LSE, MSE, MAPE, Prediction, Regression model
Introduction
Coronavirus Disease 19 (COVID-19) is a virus that became visible at the end of 2019 when it was first announced in Wuhan, China. The spreading of this new and unknown but deadly disease was very quickly and by March 11th the World Health Organization declared it as a pandemic status [1,2]. The virus had people infected in more than 114 countries with 180,000 cases and over 4000 deaths. This situation caused many schools and businesses to close, many countries decided to close their borders, and many people in different countries started living under a quarantine.
Even though, the rate of mortality among those infected is 2.3 percent, which is not high, we do not actually have a vaccine to stop its spreading worldwide which is what more frightens people. It is clear that COVID-19 is very contagious and that everyone should be held responsible for their own safety. However, it has been found that not every infected person has been admitted into hospitals and is important to identify the factors that can cause the disease to worsen. There is a little information known about this disease, but what professionals have informed is that the symptoms are fever, dry cough, chest distress among others. The study, "Prediction of Number of Cases of 2019 Novel Coronavirus (COVID-19) Using Social Media Search Index" (Qin) shows how web and social media can be used by tracking keywords, such the previous mentioned symptoms, to predict the new or suspected cases of COVID-19. This information is very helpful to prepare health institutions for possible outbreaks, to give the opportunity to governments to implement new policies as a stricter quarantine, or to educate the population living in high risk areas. This would be another method used to predict. Our analysis will focus on prediction as well for the number of death cases that will be caused by COVID-19 according to the data provided.
This paper will consider 126 countries as they have complete data for all variables and represent the world populations very nicely. We will focus on determining what variables most influence the death by COVID-19. The variables and a brief description are provided below:
Total deaths per million (Y): Testing policies differ in each country and it is not possible to use the total number of cases as a dependent variable. In this paper, the dependent variable is the number of deaths per million because of COVID-19 in each country.
Population density (X1): Population density is a measurement of population per unit area. Considering that the virus is spread from person to person, how crowded a population is may influence the transmission of the disease.
Median age (X2): Age seems to be an important factor respecting the seriousness of the illness. There were a few children hospitalized because of COVID-19 and many of older adults. The median age of a population shows where it is a young or old population.
People age 70 and older (X3): The mortality of the virus tends to increase among people who are 70 and older who are considered one of the risk groups.
GDP per capita (X4): Gross Domestic Product measures the total income of a country’s economy in US dollars. A higher GDP is related to a higher quality of life, more years of education, and better health services.
CVD death rate (X5): Pre-existing conditions may influence the seriousness of the virus. Mortality increases to a 10 percent among COVID-19 patients with previous cardiovascular diseases.
Diabetes prevalence (X6): Patients who have diabetes and may not have their blood levels controlled have a weak immune system. Therefore, it is harder for them to get rid of the virus.
Smokers-tobacco prevalence (X7): Since COVID-19 is a respiratory virus, those patients who already have their lungs and respiratory systems in poor conditions may have a hard time while fighting the disease.
Hospital beds per 100,000 people (X8): COVID-19 is a very contagious disease which infects people at a very high rate and has made hospitals collapse in some countries. How well a country is prepared to hospitalize a large number of people at the same time will not make doctors choose who to assist.
Total cases per million (X9): COVID-19 is a disease with low mortality rates but the amount of total cases may help predict the number of deaths. More cases will be related to more deaths.
Ghosal, et al. [3] consider SARS-CoV-2 at 6 weeks from day 0 data to predict the number of deaths in India. They explain that this virus has the ability to undergo genetic recombination and the susceptibility to natural selection explains why COVID-19 is spread very quickly. An effective prediction may help to prevent future catastrophes. They consider total number of infected cases, active cases, and recovery numbers, as regressors and total deaths and case fatality rates as a response variable. Lin, et al. [4] consider COVID-19 data to predict the number of cases using social media search index data. The literature on the fitting regression model to predict the total number of deaths using COVID-19 data is limited. There are many researches for various purposes available to fit multiple regression models in literature, to mention a few, Motulsky and Christopoulos [5], Montgomery, Douglas, et al. [6], James, et al. [7], and very recently Guzzman and Kibria [8] and Saleh, et al. [9] among others.
COVID-19 is a very severe type of disease and the main objective of this paper is to identify some significant variables that will contribute towards the death rate caused by coronavirus. The organization of this paper is as follows: The data and the descriptive statistics are given in Section 2. Regression models are developed in Section 3. Cross validation and evaluation of the fitted model are outlined in Section 4. This paper will end with some concluding remarks in Section 5.
Data Sources and Data Descriptions
We started data collection by extracting the publicly available data until May 29, 2020 for COVID-19 for our analysis. First, we selected the last day of data for each country and then decided to delete some islands or small countries that were missing a lot of information (in this case regressors) and finally we ended up with 126 countries with nine regressors. Then, we verified that we had countries the five continents. Those countries are Albania, United Arab Emirates, Argentina, Armenia, Australia, Austria, Azerbaijan, Belgium, Benin, Burkina Faso, Bangladesh, Bulgaria, Bahrain, Bahamas, Bosnia and Herzegovina, Belarus, Brazil, Barbados, Brunei, Botswana, Canada, Switzerland, Chile, China, Colombia, Comoros, Cape Verde, Costa Rica, Cyprus, Czech Republic, Germany, Djibouti, Denmark, Dominican Republic, Algeria, Ecuador, Egypt, Eritrea, Spain, Estonia, Ethiopia, Finland, Fiji, France, United Kingdom, Georgia, Ghana, Gambia, Greece, Croatia, Haiti, Hungary, Indonesia, India, Ireland, Iran, Iceland, Israel, Italy, Jamaica, Japan, Kazakhstan, Kenya, Kyrgyzstan, Cambodia, South Korea, Kuwait, Laos, Lebanon, Liberia, Sri Lanka, Lithuania, Luxembourg, Latvia, Morocco, Moldova, Mexico, Mali, Malta, Myanmar, Montenegro, Mongolia, Mozambique, Mauritius, Malawi, Malaysia, Niger, Netherlands, Norway, Nepal, New Zealand, Oman, Pakistan, Panama, Philippines, Poland, Portugal, Paraguay, Qatar, Romania, Russia, Saudi Arabia, Singapore, El Salvador, Suriname, Slovakia, Slovenia, Sweden, Swaziland, Seychelles, Togo, Thailand, Timor, Tunisia, Turkey, Tanzania, Uganda, Ukraine, Uruguay, United States, Uzbekistan, Vietnam, Yemen, South Africa, Zambia, and Zimbabwe.
For this study we consider the following variables: total deaths per million (Y), population density (X1), median age (X2), people age 70 and older (X3), GDP per capita (X4), CVD death rate (X5), diabetes prevalence (X6), smokers-tobacco prevalence (X7), hospital beds per 100,000 people (X8), and total cases per million (X9). Table 1 shows the descriptive statistics for the dependent and independent variables. The range for the number of deaths because of COVID-19 per million is large which means that the virus did not attack every country the same way.
The objective of this study is to determine if any of the nine regressors influence the number of deaths because of COVID-19. In order to determine if any of the factors are significant, we will construct a multiple linear regression model that relates the number of deaths to the nine regressors in the section follow.
Statistical Analysis
We will do the regression analysis in this section. We will consider the top 80% of our data (approximately the first 101 countries). The 20% at the bottom will be used later to evaluate the adequacy of the linear regression model. Therefore, the sample size of 126 was reduced to 101.
Now, we will consider the following linear regression model:
y = β0 + β1x1 + β2x2 + β3x3 + β4x4 + β5x5 + β6x6 + β7x7 + β8x8 + β9x9 + ε (1)
where, y = total deaths because of COVID-19 per million, x1 = population density, x2 = median age, x3 = people age 70 and older, x4 = GDP per capita, x5 = CVD death rate, x6 = diabetes prevalence, x7 = smokers- tobacco prevalence, x8 = hospital beds per 100,000 people, and x9 = total cases of COVID-19 per million. In order to fit the model, we will assume that all regressors are independent and that the residuals are normally distributed with mean 0 and variance σ2.
After fitting a regression model, from SPSS we get the results shown in Table 2.
Using Table 2, the first full fitted model is:
y = 19.808 + 0.011x1 + 2.682x2 + 12.602x3 - 0.001x4 - 0.177x5 - 6.218x6 - 0.282x7 - 14.044x8 + 0.025x9 (2)
We obtain the value of R Square as 0.423 (adjusted R Square 0.37), which means that almost 45% in total variation of deaths has been explained by the nine variables. We can see from Table 2 that some of the regressors are not significant for the model.
The normal Q-Q plot and Residuals vs. Fitted plot are shown in Figure 1 and Figure 2 respectively.
We can see from Figure 1 that the residuals are approximately normal, while Figure 2 shows that the constant variance assumption has not been met.
In order to get an adequate model, we have tried various transformations on the dependent variable (Y). However, the log of Y transformation gave the better model, which is stated below.
y* = log (Y) = β0 + β1x1 + β2x2 + β3x3 + β4x4 + β5x5 + β6x6 + β7x7 + β8x8 + β9x9 + ε (3)
The normal Q-Q plot (Figure 3) shows how the data now follows a normal distribution. Also, the Residuals vs. Fitted plot (Figure 4) shows a scatter plot distributed approximately even around 0. This indicates that the constant variance assumption has been satisfied. The regression analysis by SPSS for transformed model is provided in Table 3.
Using Table 3: The transformed fitted model is given below:
y = -1.025 + 0.000x1 + 0.067x2 + 0.039x3 + 0.000x4 + 0.000x5 - 0.004x6 - 0.008x7 - 0.067x8 + 0.000x9 (4)
We obtain the value of R Square as 0.587 (Adjusted R Square 0.544), which means that almost 60% in total variation of deaths has been explained by the nine variables. Since the F-test statistic is 13.600 and its corresponding p-value is 0.000, we can reject the null hypothesis that the regressors are not significant. Therefore, we can assume that at least one variable is significant to the model. Now, we would like to reduce the model by backwards elimination. Using SPSS, we come with the following reduced model (R2 = 0.576) Table 4.
We have decided that, even though the p-value for X7 in Table 5 is greater than 0.05, we will keep it in the model. Variable X7 is tobacco prevalence and has a relationship with deaths by COVID-19 [10]. Our model resulting from regressor elimination:
y = -0.919 + 0.054x2 + 0.057x3 - 0.008x7 - 0.069x8 + 0.000x9 (5)
Our final model has only five variables left (x2, x3, x7, x8, and x9) that are significant with the deaths by COVID-19 (R2 = 0.581).
Multicollinearity
Some assumptions need to be met in a multiple linear regression model and one of them is that variables should be independent from each other. This means that there should not be any relationship between the regressors. To check if there is no multicollinearity in our model, we will check Table 5. The VIF for x2, x3, x7, x8, and x9 are 7.130, 5.819, 1.648, 2.120, and 1.239 respectively. We can see that the VIF for all variables are less than ten, which means that multicollinearity is not a problem.
Cross Validation
Cross Validation is used to justify whether a model is adequate or not. In this case, we are selecting the last 20% of our data, from 102 to 126 to predict the corresponding y values using cross validation. To justify its accuracy, we will determine which model would be best suited for prediction. Using this test set, we will compare those predicted values to their corresponding original values. If there are slight differences, it can be said that the model is adequate enough to predict future accurate results. We will consider the last two models, one with variables x2, x3, x7, x8, and x9 and the other without x7. Then we calculate the following statistics: Mean Absolute Error (MAE), Root Mean Square Error (RMSE), Range-normalized RMSE (NRMSE), and Mean Absolute Percentage Error (MAPE) to compare the performance of the model.
For model 3.4 with variables x2, x3, x7, x8, and x9 we get:
MAE = 0.34819
RMSE = 0.45537
NRMSE = 0.00108
MAPE = 0.31924
For model 3.5 with variables x2, x3, x8, and x9 we get:
MAE = 0.35409
RMSE = 0.47142
NRMSE = 0.00112
MAPE = 0.31710
The MAE, RMSE, and NRMSE are a little bit higher in the second model which indicates that the first model is better for our data. Thus, the final model would be:
Log (y) = -0.919 + 0.054x2 + 0.057x3 - 0.008x7 - 0.069x8 + 0.000x9
Using the above final model, we will predict the total number of deaths of COVID-19 per million for the last 24 countries and provided them in Table 6.
From Table 6, it appears that the difference between the predicted and the original number is not very large. Therefore, we can conclude that our model predicts the total number of deaths per million pretty accurately.
Concluding Remarks
This paper considers developing a predictive model for the total deaths of COVID-19 per million citizens data. There were considered nine regressors: population density, median age, people 70 and older, GDP per capita, CVD death rate, diabetes prevalence, tobacco prevalence, hospital beds per 100k people, Total cases of COVID-19 per million in the model. After fitting a full model, a transformed model, and a reduced model using backward elimination, we concluded that only five variables were significant when analyzing the deaths because of COVID-19. Those variables were median age (2), people 70 and older (3), tobacco prevalence (7), hospital beds per 100k people (8), and total cases of COVID-19 per million (9). This paper considers COVID-19 data until May 29, 2020. However, one can extend this paper with updated data with the same or different models.
Acknowledgements
Authors are thankful to three referees for their valuable comments and suggestions, which certainly improved the presentation and quality of the paper. They wish to dedicate this paper to those who have lost their lives due to COVID-19 in USA.